Accurate precipitation forecast, especially heavy precipitation forecast, is challenging in weather forecasts. Heavy precipitation always brings about flash floods, urban waterlogging, heavy snow, and other disasters, making it imperative to improve the forecast performance of precipitation, especially heavy precipitation. Certain bias is still found in current precipitation forecast. For example, the forecast performance of winter precipitation is far lower than that in summer in Southeastern China. To address this problem, Huang Wenyu’s Research Group of DESS, Tsinghua University proposed a deep learning-based winter precipitation bias correction method, improving the precipitation and heavy precipitation forecast performance.
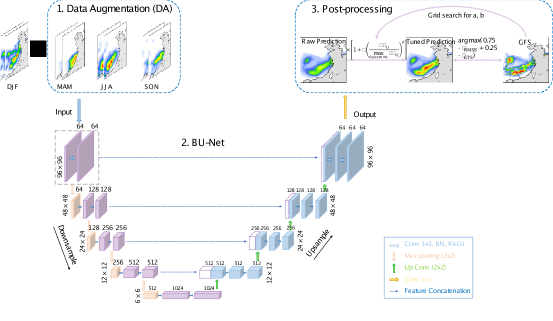
Fig. 1 The DABU-Net model architecture.
This research uses the Global Forecast System (GFS) forecast data as input and the precipitation of forecast lead times of 24, 48, and 72 hr, and 13 other relevant variables as input variables of the neural network to build three independent models for the forecast lead times of 24, 48, and 72 hr, respectively. The precipitation observation data is the gridded product of the Climate Prediction Center (CPC) integrating satellite and ground observations. The CPC precipitation data is further bilinearly interpolated to the resolution of the GFS forecast data with a uniform resolution of 0.25°. The bias correction model DABU-Net consists of three modules (Fig. 1) , data augmentation (DA) module, the neural network BU-Net module and post-processing module. Data enhancement mainly uses the samples of three other seasons as the enhanced sample set of winter precipitation to solve the problem of less extreme precipitation samples caused by unbalanced distribution of winter precipitation. BU-Net is a U-Net with batch normalizations, consisting of down-sampling, up-sampling and skip connections. The combination of its encoder and decoder can well extract spatial features of different scales from images. The main purpose of post-processing is to bring TS scoring index which is more closely related to extreme precipitation into the model and further improve the forecast of extreme precipitation. The deep neural network is trained for three lead times and its simulation performance on the test set is verified.
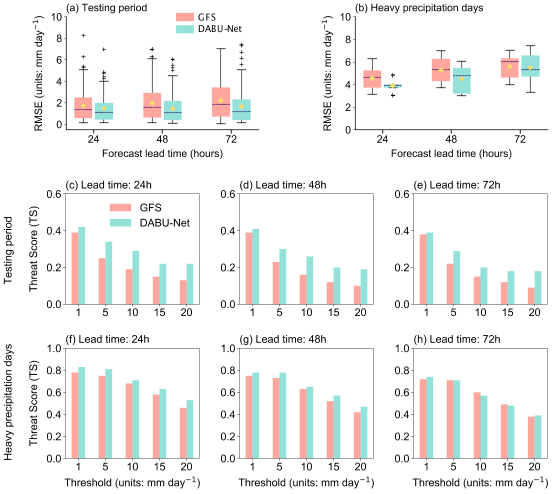
Fig. 2 Box-and-whisker diagrams summarizing the distributions of the Root Mean Squared Errors
During the testing period, the RMSEs of the GFS forecast are 1.73, 1.96, and 2.19 mm day−1 for the three lead times, respectively. After using DABU-Net, the RMSEs are reduced to 1.40, 1.47, and 1.70 mm day−1 at the three lead times, corresponding to percentage decreases of 19.08%, 25.00%, and 22.37%, respectively. In particular, TS at the threshold of 20 mm day−1 are increased by 69.23%, 90.00%, and 100.00% at lead times of 24, 48, and 72 hr., demonstrating its forecast performance of heavy precipitation (Fig. 2). The precipitation forecast biases corrected by the use of DABU-Net are obviously reduced in the whole region. At the lead time of 24 hr, the largest RMSEs of precipitation are located in Hunan and Guangxi provinces, which are larger than 5 mm day−1 . The RMSEs over the above areas are reduced to less than 3.5 mm day−1 after using DABU-Net. During heavy precipitation days exceeding 5mm/day in the winter of 2021-2022, the precipitation RMSEs forecasted by GFS at the three lead times are 4.56, 5.27, and 5.59 mm day−1, respectively. After using DABU-Net, the precipitation RMSEs in SEC are reduced to 3.91, 4.52, and 5.50 mm day−1. Correspondingly, the percentage decreases in precipitation RMSEs reach 14.25%, 14.23%, and 1.61%, respectively. For the 20 mm day−1 threshold, the TS at the three lead times increase by 15.22%, 11.90%, and 2.63%, respectively, demonstrating that the DABU-Net still works under heavy precipitation conditions at the lead times within 48 hr. It can be therefore concluded that the winter precipitation prediction approach based on deep neural network for Southeastern China is very effective.
The relevant research has been published in the form of a paper titled “Deep Learning Improves GFS Wintertime Precipitation Forecast Over Southeastern China in Geophysical Research Letters. Sun Dan, a doctoral student of Class 2019 of the DESS, Tsinghua University is the first author, and his supervisor Huang Wenyu is the corresponding author. Collaborators are from the DESS, Tsinghua University and the Nanjing University of Information Science and Technology.
Full-text link of the paper: https://agupubs.onlinelibrary.wiley.com/doi/full/10.1029/2023GL104406
Written by Sun Danyi
Edited by Wang Jiayin
Reviewed by Zhang Qiang