Accurate offshore surface wind forecasting is crucial for navigation safety and disaster prevention. Sea surface roughness is a key factor affecting offshore windsite forecasting. However, significant biases exist in forecasting sea surface winds due to the uncertainties in estimating sea surface roughness. However , traditional methods for calculating sea surface roughness, which primarily relied on simple regression algorithms and in situ observation data on atmospheric conditions and waves to develop parameterization schemes, have obvious limitations in capturing the complex nonlinear relationships among atmospheric and wave parameters related to sea surface roughness. Due to the uncertainty of the estimated sea surface roughness, the forecast of sea surface wind is biased. As deep learning method can capture the complex nonlinear relationship in a large number of data, it merits our efforts to explore whether deep learning method can be used to develop a new parameterization scheme of sea surface roughness.
To address the above problem, Huang Wenyu’s Research Group of the Department of Earth System Science (DESS), Tsinghua University uses the Air-Sea Interaction Tower (ASIT) of the Northwest Pacific Ocean to propose a sea surface roughness scheme based on deep learning. Single-point experiments demonstrate that DL2023 achieves a remarkable 50% reduction in the Root Mean Square Error (RMSE) compared to the four traditional schemes (Fig. 1).
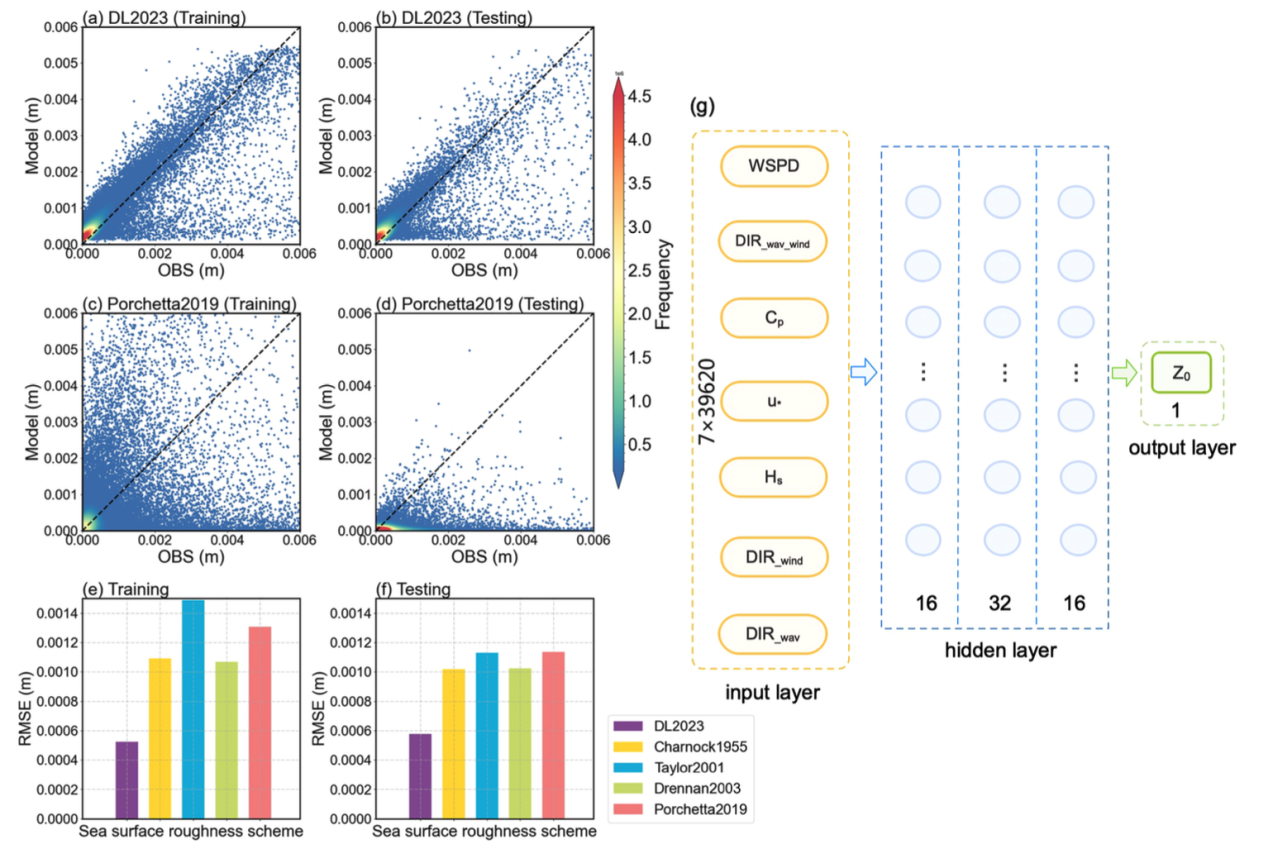
Fig. 1 Density scatter plots of the sea surface roughness (units: m) forecasted by (a–b) DL2023 and (c–d) Porchetta2019 on the training and testing data (y-axis) against observation (x-axis). Root Mean Squared Errors (RMSEs; units: m) of sea surface roughness between the forecast using five sea surface roughness schemes and observation on the (e) training and (f) testing data. (g) Schematic diagram of deep neural network for the deep learning-based sea surface roughness scheme.
To investigate whether this approach can improve the model's accuracy in forecasting sea surface winds, the Research Group has integrated this scheme into a regional ocean-atmosphere-wave coupled model in the Northwest Pacific Ocean (NWPO) region. During five typhoon cases in August 2020, compared to the four traditional schemes, the RMSEs of forecasted surface winds using DL2023 are reduced by 6.02%–14.75%, 11.17%–18.30%, and 11.91%–19.46% at lead times of 24, 48, and 72 hr, respectively (Fig. 2). Thus, the DL2023 scheme successfully improves the forecast of surface winds over the Northwest Pacific Ocean.
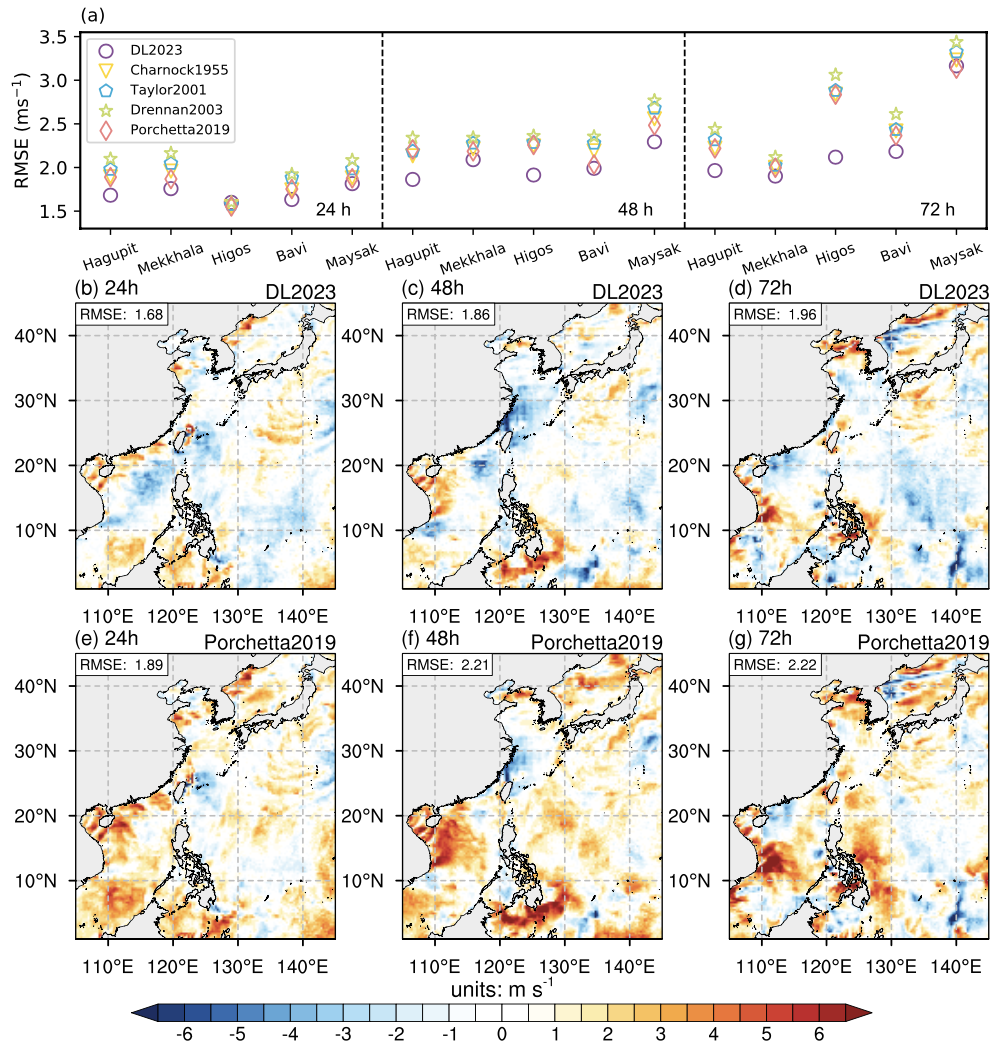
Fig. 2 (a) Root Mean Squared Errors (RMSEs; units: m s−1) of surface wind speed at 10 m height of the coupled model using DL2023 (purple circle), Charnock1955 (gold triangles), Taylor2001 (blue pentagons), Drennan2003 (green stars), and Porchetta2019 (pink diamonds) at lead times of 24 hr (left), 48 hr (middle), and 72 hr (right) during the five typhoons (x-axis) in August 2020. (b–g) Horizontal distribution of the bias of the forecasted surface wind speeds (units: m s−1) by DL2023 and Porchetta2019 with respect to the observation at the lead times of 24 hr (left), 48 hr (middle), and 72 hr (right) during the typhoon Hagupit.
The above work has innovatively realized the application of deep neural networks in the parameterization of sea surface roughness. It is noteworthy that the data used for deep learning-based sea surface roughness parameterization scheme are from an observation tower in the North Atlantic Ocean, while the successful application of the scheme in the Northwest Atlantic Ocean indicates the strong transferability of the new scheme.
The related research results have been published in the form of a paper titled “Deep Learning-Based Sea Surface Roughness Parameterization Scheme Improves Sea Surface Wind Forecast” in Geophysical Research Letters. Fu Shu, doctoral candidate of Class 2020, of the DESS, Tsinghua University, is the first author of the paper, and Associate Professor Huang Wenyu of the DESS, Tsinghua University is the corresponding author. Other collaborators of the paper are from Tsinghua University, Nanjing University of Information Science and Technology, and Beijing Forestry University. This work was jointly supported by National Key R&D Program of China (2020YFA0608000), National Natural Science Foundation of China (42275154), and Tsinghua University Initiative Scientific Research Program (2019Z07L02011).
Paper information:
Fu, S., Huang, W., Luo, J., Yang, Z., Fu, H., Luo, Y., & Wang, B. (2023). Deep learning-based sea surface roughness parameterization scheme improves sea surface wind forecast. Geophysical Research Letters, 50, e2023GL106580. https://doi.org/10.1029/2023GL106580
Full-text link: https://doi.org/10.1029/2023GL106580
Written by Fu Shu
Edited by Wang Jiayin