Land surface process is a key process in Earth’s surface system, and accurate estimation of land surface state plays an important role in improving the performance of an Earth system model. The Land surface data assimilation system improves the observability and predictability of land surface state variables by integrating the advantages of multi-source Earth observation data and land surface models. The uncertainty of parameters in land surface models and the lack of effective estimation methods of model errors and observation errors in the assimilation system have hindered the further development and popularization of the assimilation system. Therefore, it is imperative to simultaneously estimate multiple parameters and errors in data assimilation system with high efficiency and coordination.
To address the above problem, Professor Lu Hui’s Research Group of the Department of Earth Science System (DESS), Tsinghua University has developed a dual-cycle assimilation algorithm based on Ensemble Kalman filter (EnKF), resolving the difficulty of simultaneous estimation of error parameter, model parameter and observation operator parameter. However, the low-efficiency parameter estimation of the dual-cycle algorithm limits its application potential in large-scale assimilation research. To address this issue, the authors replaced the EnKF with the EKF based on the automatic differentiation (AD) method to reduce the model set’s cost of parameter estimation, realizing the high-efficiency estimation of multi-parameters in data assimilation system. To validate the EKF-based dual-cycle algorithm, two groups of experiments were designed in the study, single point assimilation experiment DA_EKF and DA_EnKF (experiment 1) and assimilation experiment DA_TP and simulation experiment OL_TP (experiment2) over the Tibetan Plateau.
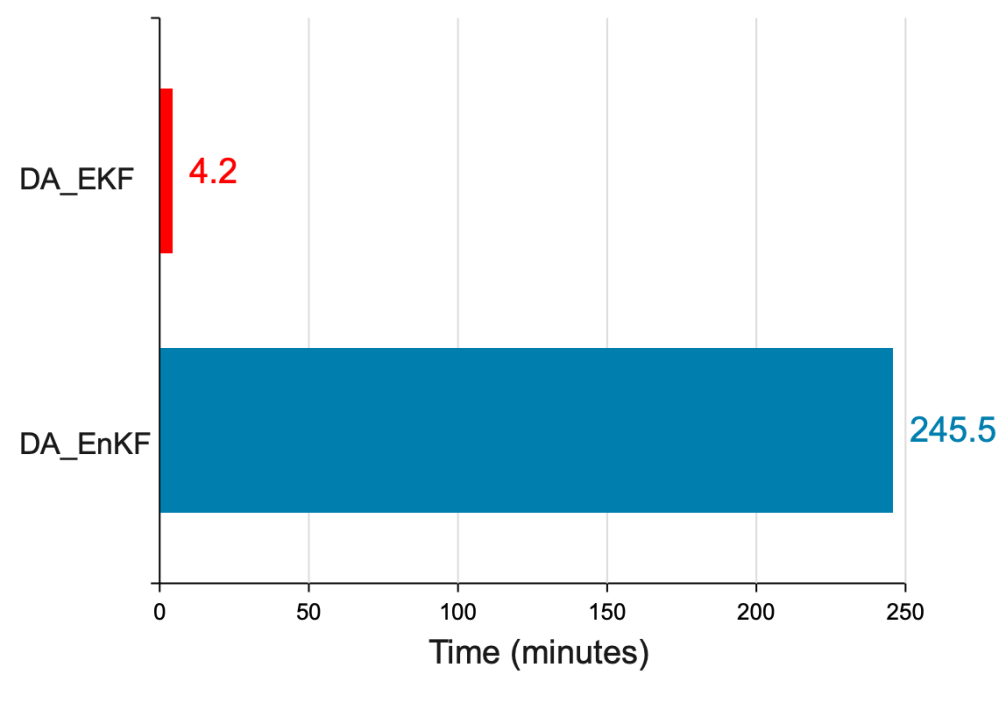
Figure 1 Time consumption of parameter optimization time (minutes) for dual-cycle LDAS based on EKF and EnKF
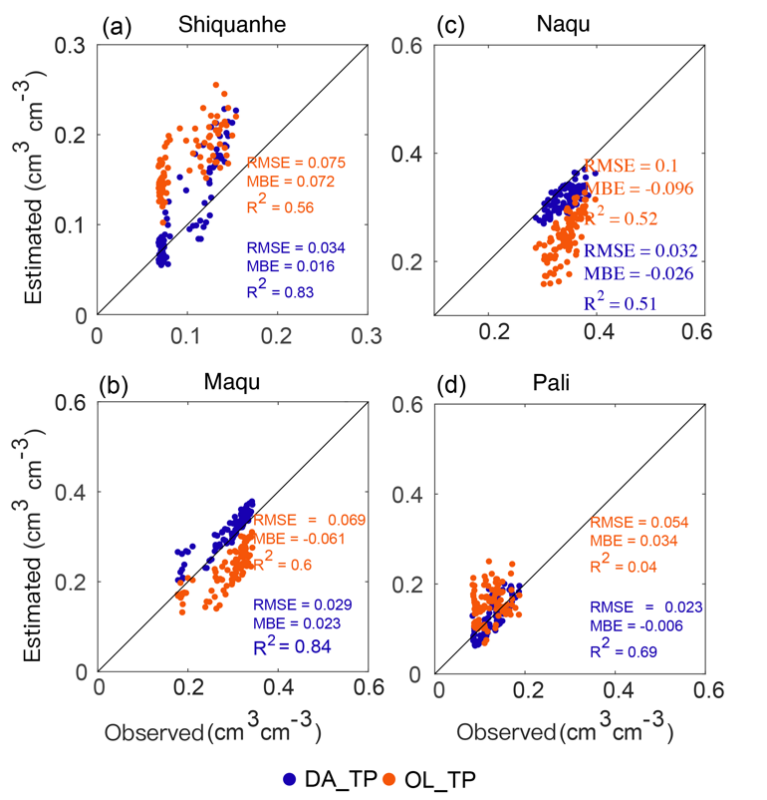
Figure 2 Scatter plots of soil moisture from TP_OL, TP_DA, SMAP and in-situ measurement from (a) WTP Shiquanhe, (b) CTP-Naqu, (c) ETP-Maqu, and (d) STP-Pali regions
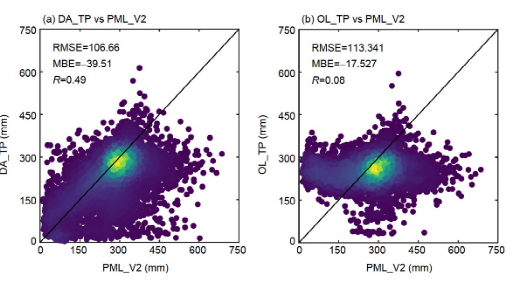
Fig. 3. Scatter plots of PML_V2 ET against (a) DA_TP and (b) OL_TP
It has been found in the research that the EKF-AD-based dual-cycle algorithm accelerated the parameter estimation efficiency near 60 times (Fig. 1). In the Tibetan Plateau assimilation experiment, the estimation error of soil moisture is obviously smaller than that of the default parameter simulation experiment (Fig. 2). In addition, through validation, it has been found that the assimilation of soil moisture also has a certain positive effect on evapotranspiration simulation (Fig. 3).
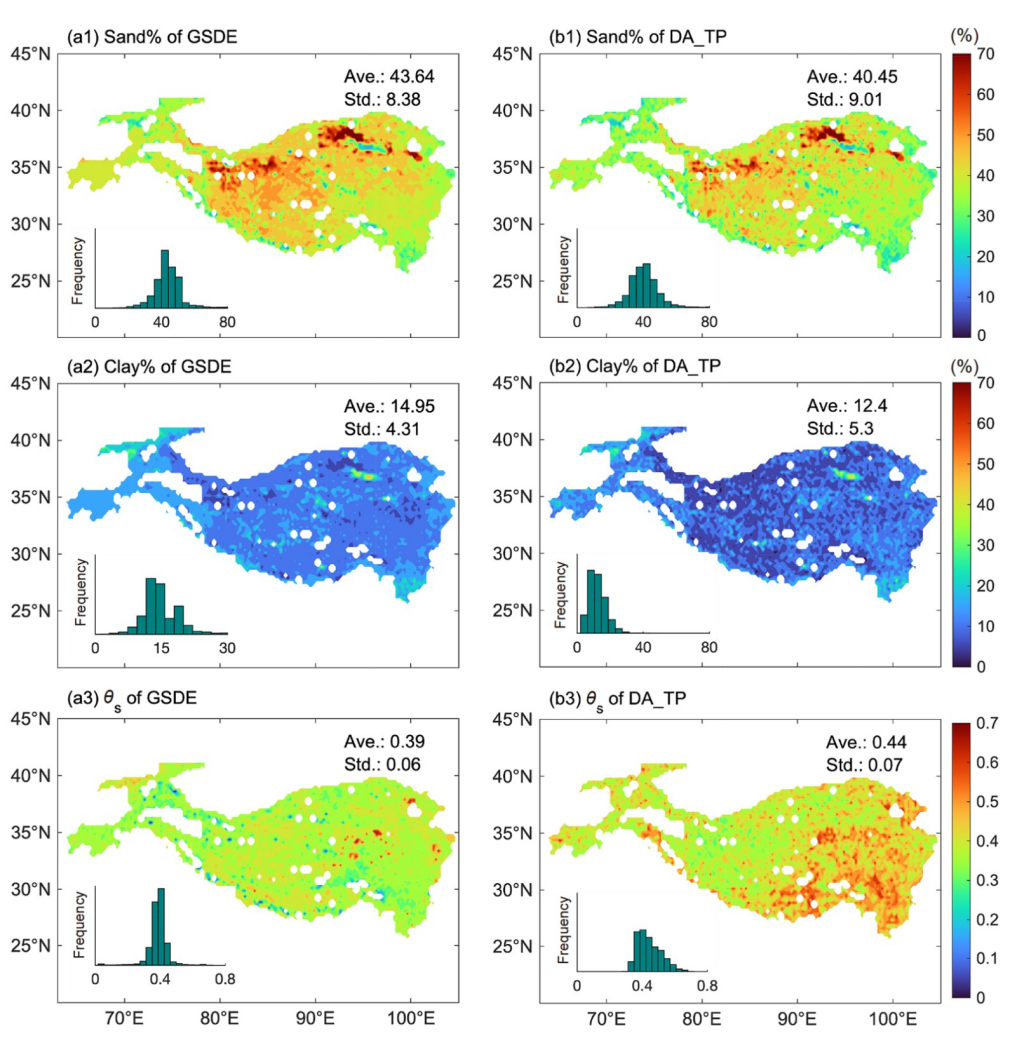
Fig. 4 Maps of soil parameters from GSDE and DA_TP. (a1) and (b1) sand content, (a2) and (b2) clay content, (a3) and (b3) soil porosity
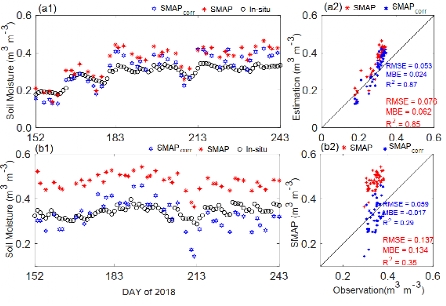
Fig. 5 Time series and scatter plots of soil moisture from SMAP and SMAP_corr of (a) Naqu and (b) Maqu observation network, and in-situ measurement.
The EKF-based dual-cycle algorithm can simultaneously estimate model parameters, observation operator parameters and error parameters. Through comparison, it has been found that the soil parameter estimates of the assimilation system was more reasonable than that of the default values of land surface models (Fig. 4). In addition, optimized observation operator parameters can be used to correct the bias of SMAP soil water content products (Fig. 5).
The EKF-based dual-cycle LDAS demonstrates a promising assimilation performance in regional scale experiments on the Tibetan Plateau, providing potential new insights for hydrological, ecological, and land data assimilation studies. The related research results have been published in Science China Earth Sciences in the form of a paper titled “Quick estimation of parameters for the land surface data assimilation system and its influence based on the extended Kalman filter and automatic differentiation”. In addition, the Research Group has used the dual-cycle data assimilation system to improve soil content simulation through assimilating satellite land surface temperature observations. The research results have been published in IEEE Journal of Selected Topics in Applied Earth Observations and Remote Sensing in the form of a paper titled “Improving Surface Soil Moisture Estimation Through Assimilating Satellite Land Surface Temperature With a Linear SM-LST Relationship”.
Postdoctoral Fellow Tian Jiaxin of the DESS, Tsinghua University is the first author of the paper, and Professor Lu Hui is the corresponding author of the paper. Co-authors are from the DESS, Tsinghua University, the Institute of Geographic Sciences and Natural Resources Research, Chinese Academy of Sciences, and South-west University. This work was supported by the Second Tibetan Plateau Scientific Expedition and Research Program (Grant No. 2019QZKK0206), the National Key Research and Development Program of China (Grant No. 2022YFC3002901), and the National Natural Science Foundation of China (Grant No. 42271491).
Paper information:
Tian, J., Lu, H., Yang, K. et al. Quick estimation of parameters for the land surface data assimilation system and its influence based on the extended Kalman filter and automatic differentiation. Science China Earth Sciences. 66, 2546–2562 (2023). https://doi.org/10.1007/s11430-022-1180-8
Tian, J., Lu, H., Yang, K. et al. Improving Surface Soil Moisture Estimation Through Assimilating Satellite Land Surface Temperature with a Linear SM-LST Relationship. IEEE Journal of Selected Topics in Applied Earth Observations and Remote Sensing. 16,7777-7790 (2023). http://doi.org/10.1109/JSTARS.2023.3305888
Written by Tian Jiaxin
Edited by Wang Jiayin
Reviewed by Lu Hui and Zhang Qiang