报告题目:Understanding moist convection-environment interaction from high-res modeling and observations
主讲人:Yang Tian(田阳) 研究员
美国国家大气研究中心(NCAR)
时间:2022年3月10日(周四) 10:00─11:30
地点:清华大学蒙民伟科技大楼南楼S820
腾讯会议:440-226-418
讲座简介:
In this talk, I will be primarily focused on two related topics: 1). What controls the turbulent mixing between clouds and environment? – One vital yet most uncertain process in convection representation. A novel Lagrangian analysis framework embedded within Large Eddy Simulation is used to probe into the underlying mechanism, and a new entrainment formula is proposed. Using the similar framework, a deterministic model is proposed to better represent updraft vertical velocity in convection scheme as well; 2). What controls the transition from shallow to deep convection? The insufficient understanding of which has induced a major drawback in reproducing the diurnal cycle of precipitation. A suite of airborne, ground-based and satellite observations is incorporated into a plume model to disentangle the relative contribution from surface and atmosphere, and reveal possible pathways underlying future droughts/floods.
主讲人简介:
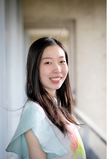
I am an atmospheric scientist who is particularly drawn to the dynamics of moist convective systems and their interactions with the large-scale environment, such as entrainment process, convective sensitivity to environmental perturbations etc. This interaction is key to the precipitation distribution and variability, as well as Earth’s radiation budget, but is poorly comprehended and simulated. In May, 2018, I received my doctoral degree in Earth & Planetary Sciences from Harvard University, advised by Zhiming Kuang. After graduation, I have been working with Steve Klein and Yunyan Zhang at LLNL on cloud processes over the Amazon region. As a scientist at NCAR, I am using both high-resolution models and observations to further validate and improve convection representation in CAM7, as well as investigating other highly coupled systems (e.g. land-atmosphere) for next-generation Earth System Models.