The solar photovoltaics (PV) industry has grown rapidly, raising very high requirements on high-quality solar radiation data (including total radiation data and Rdir and Rdif component data). Satellite-based remote sensing data inversion is the main means to obtain total radiation data. However, due to the inhomogeneity of time series caused by satellite replacement and technical iteration, the inversion results can hardly reflect the long-term trend of radiation change. In addition, due to the lack of surface observation, it is challenging to construct a reliable estimation model of direct and diffuse radiation components, that is, a high-quality gridded dataset. With regard to the above problem, Professor Yang Kun’s Research Group of the Department of Earth System Science (DESS), Tsinghua University has developed a global study, a convolutional neural network-based homogenization method is developed to use a reanalysis dataset (ERA5) as a bridge to homogenize a 36-year (1983–2018) satellite-based Rs dataset (so-called ISCCP-ITP) with 10-km spatial resolution and 3-hr interval, and a 36-year (1983 to 2018), 10-km daily Rdif and Rdir dataset over China.
As for the total radiation data, the spatial and temporal resolution of ISCCP-ITP total radiation dataset based on ISCCP satellite cloud data inversion is high, which can well reflect the radiation change after the year 2000, but it is overestimated compared with the ground observation data (black line in Figure 1) of Global Energy Balance Archive (GEBA) before 2000 (blue line in Figure 1). The quality of reanalysis radiation data of ERA5 is relatively uniform, and its changing trend (green line in Figure 1) is close to that of observation (black line in Figure 1), but it is obviously overestimated. According to the above analysis, this study uses the deep learning algorithm Unet, and uses the uniform reanalysis data ERA5 as a bridge to homogenize the satellite inversion data of ISCCP-ITP, thus eliminating its systematic overestimation of radiation from 1983 to 1999 (red line in Figure 1). The radiation trend of homogenized dataset ISCCP-ITP-CNN in all major regions of the world is close to that of surface observation, which has obvious advantages in accuracy compared with the international mainstream radiation dataset (Figure 2).
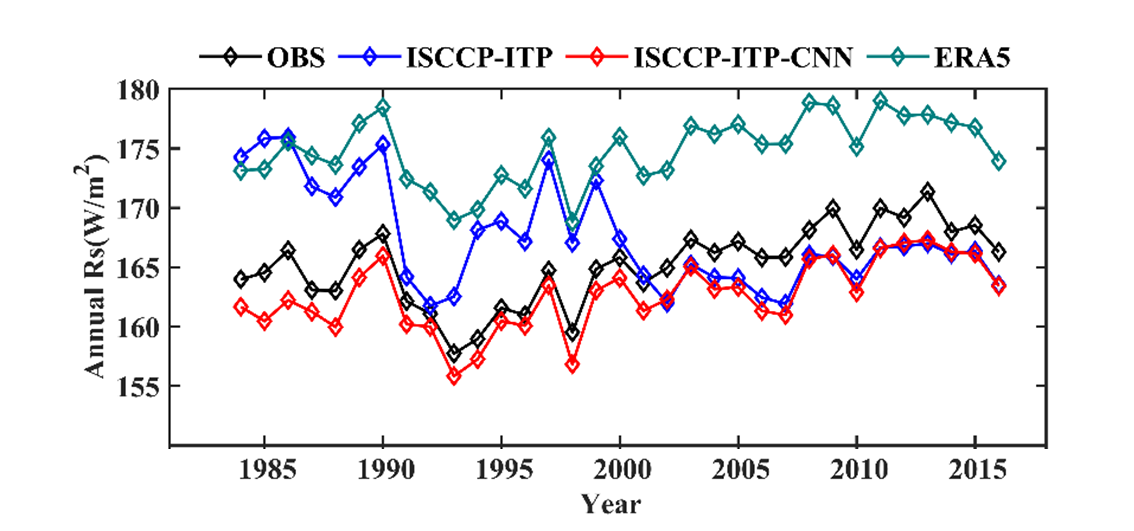
Figure 1 The global mean of the annual anomaly of the Rs (units: W/m2) during 1984–2016 derived from GEBA stations observations (in the black line), collocated grid data of ISCCP-ITP (in the blue line) and ISCCP-ITP-CNN (in the red line), and comparison of ERAs reanalysis data sets.
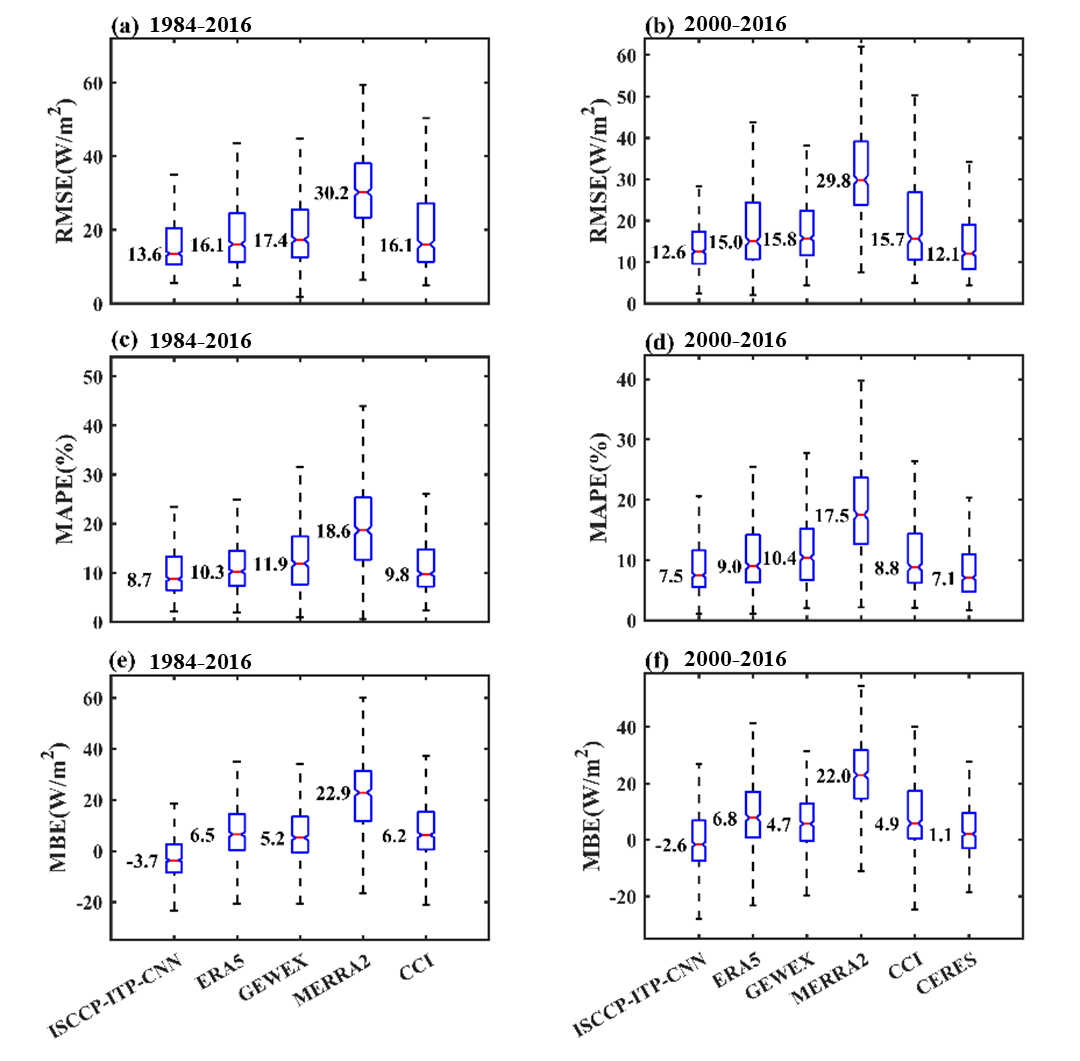
Figure 2 Comparison of (a) RMSE, (c) MAPE and (e) MBE for monthly ISCCP-ITP-CNN, ERA5, MERRA2, GEWEX-SRB (GEWEX), and Cloud_cci (CCI) during 1984–2016 and (b) RMSE, (d) MAPE and (f) MBE for monthly ISCCP-ITP-CNN, ERA5, GEWEX-SRB (GEWEX), MERRA2, Cloud_cci (CCI) and CERES-EBAF (CERES) during 2000–2016 against GEBA observations. RMSE, MAPE and MBE represent root mean square error, mean absolute percentage error, and mean bias error. The number near the box represents the median of the metrics.
For radiation component data, the current mainstream radiation estimation methods are empirical methods, calling for a large number of observed true values of radiation components to fit or train the model, but China is very short of observed data of radiation components. In this study, the sunshine hours widely observed by the Meteorological Administration were used instead, and the radiation component data were estimated based on the parameterized scheme, which augmented the training data. The data can be used as a data source for data augmentation because the amount of estimated radiation component data is huge, the data quality changes smoothly with time, and the error is random in space. Based on this dataset, this study used the LightGBM model to develop an estimation model of radiation components, which was independently verified in Chinese and global stations, and then the daily solar radiation dataset in China was built. The estimation of radiation components using CMA’s radiation observations indicated that the RMSE of daily average Rdif and Rdir is 21.3 and 32.8 W/m2 respectively (Figures 3a and 3b) . At the monthly scale, the RMSE of Rdif and Rdir are 10.5 W/m2 and 18.6 W/m2 respectively (Figures 3c and 3d). Its accuracy is significantly better than the international mainstream satellite-based and reanalysis datasets, and it also presents obvious advantages compared with the datasets based on the observation of surface radiation components, which proves the effectiveness of the data augmentation strategy adopted in this study.
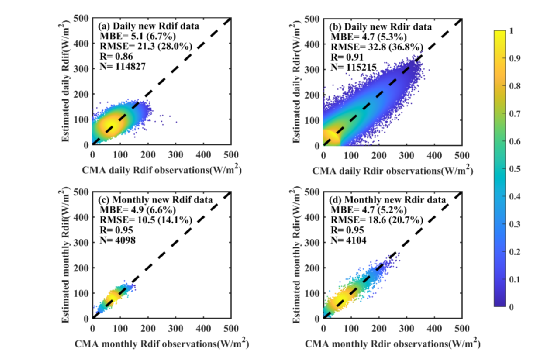
Figure 3. Evaluation for daily Rdif (a) and Rdir (b) and monthly Rdif (c) and Rdir (d) from the new dataset developed in this study during 1994 to 2015 against observations at 17 CMA stations. In the figure, MBE, RMSE, R and N represent mean deviation, root mean square error, correlation coefficient and number of scattered points respectively, and the percentage in brackets is relative error. The evaluation period is from 1994 to 2015.
The related research results have been published in Renewable and Sustainable Energy Reviews and Journal of Remote Sensing. Doctoral student Shao Changkun of Class 2020 of the DESS, Tsinghua Univer is the first author of the papers, and his supervisor Professor Yang Kun is the corresponding author. The collaborators include Researcher Tang Wenjun from the Institute of Tibetan Plateau Research, Chinese Academy of Sciences and some other teachers and students from the DESS, Tsinghua University. This work was supported by the National Natural Science Foundation of China.
Paper Information”
Shao, C., Yang, K., Tang, W. et al. (2022). Convolutional neural network-based homogenization for constructing a long-term global surface solar radiation dataset. Renewable and Sustainable Energy Reviews, 169, 112952.
Shao, C., Yang, K., Jiang, Y. et al. (2024). Data augmentation-based estimation of solar radiation components without referring to local ground truth in China. Journal of Remote Sensing, 4, 0111.
Written by Shao Changkun
Edited by Wang Jiayin
Reviewed by Zhang Qiang